Why Managing Nuance Matters for Red Teams
Bottom Line Up Front
-
An analogy borrowed from marketing helps us understand one key difference in the thinking between sophisticated adversaries and many of the red teams that try to simulate them.
-
Ad-tech is natsec-tech. Concepts that power personalized search engines and social media platforms can be used to reduce particular challenges red teams have faced in recent years.
-
Market forces seem to distort the ability of enterprises to recognize the intentions and capabilities of apex adversaries. Concepts from social sciences can help understand how.
Contents
- Introduction
- Common Challenges for Commercial Red Team Operators
- From Broadcasting to Narrowcasting
- Applications for Optimized Recommendations
- Misaligned Incentive Structures
- Takeaways
Introduction
This post doesn’t claim anything novel and the discourse here is lower quality than how these topics are discussed elsewhere. I hope the material presented stimulates interest, discussion, and differences of opinion that are conveyed constructively. It’s through the conflict and interaction of viewpoints that we can evolve our understanding of these topics and improve discourse quality.
With that said, I’d like to convey the following to a commercial audience:
-
Provide another lens to help recognize evolution in tradecraft. Many consulting red teams continue to adopt a style of tradecraft that is closer to the traditional broadcasting era, and sophisticated adversaries today continue to adopt and refine a narrowcasting approach. Under a useful level of abstraction, marketing campaigns and cyber operations are indistinguishable.
-
What it takes to build present-generation capabilities that facilitate narrowcasted communications has very little to do with what it takes to build “malware” and “C2”. Much like what it took to build the computational devices used to decrypt Enigma messages in WWII had very little to do with what it took to build artillery used on the battlefield. Yet the analysis from the decrypted texts had a lot to do with how the artillery was positioned.
-
There are myopic and self-reinforcing incentive structures in the commercial space that slow down our ability to make (or even recognize) sophistication leaps—unless they can be positively rewarded by market forces. This is a constraint that the sophisticated adversaries we are trying to defend against do not have to contend with in their efforts for innovation.
In other words, we’ll use a mental model from marketing to understand how tradecraft can evolve, understand why ad-tech is relevant to red team tooling, and briefly describe what makes commercial InfoSec slow to adapt to advances in apex adversary workflows.
If this sounds like your cup of tea, then grab it and start sipping. It’s going to be another dense read. While I encourage you to read through it all, it doesn’t have to be done in the same sitting. Take your time and don’t feel rushed.
Common Challenges for Commercial Operators
I’m going to start with outlining three areas that appear to be especially challenging to commercial red team operators in recent years. Of course, this is subjective and there are other ways to frame challenges. I assert these ones only as an outcome of the perspectives I’ve sought.
-
Ever-growing complexity and cognitive load on “when to use what”. Documentation media used by most red teams today to guide tradecraft decisions (wikis, runbooks, etc.) simply cannot adapt fast enough to the rapid and increasingly nuanced defensive developments that affect the eligibility of “courses of action” (CoAs). When guidance isn’t updated fast enough, or in a way that easily accomodates for new wrinkles to consider, this makes it harder to make decisions on when and how to use a particular TTP. Decisions that are scaled yet tailored for specific situations cannot rely on data that is only structured for human consumption.
-
Expensive human decisions are being used to counter cheap machine decisions. Think about the hours/days it could take to research and prepare TTPs, and how quickly that effort could be for naught when a course of action is detected in mere seconds. Going back to the Enigma analogy, the demoralizing sentiment is slightly similar to the time pressure involved in earlier manual decryption efforts—only to have the cipher periodically reset with asymmetric ease. In the EDR age, valuable operator time is increasingly spent discovering and exploiting vulnerabilities in machine decision-making than in human decision-making.
-
Tradecraft expressions lack enough diversity to be considered amorphous. As defensive sensors continue to improve, the “shape” of what Red Team TTPs look like become easier for defenders to proactively discern. Amorphous expressions deny defenders the ability to easily analyze and reason about them (e.g. as a coherent object taking up contiguous space when using visualization techniques). This diversity challenge isn’t just about limitations in the quantity of tactical capabilities, but also limitations in the manner in which they are used or not used as they relate to the circumstances at hand.
What a weapon can accomplish in time of war is not merely a function of what is technically feasible. … A weapon's capability is dependent to some extent on being correctly "found," that is, being chosen and utilized in the proper circumstances in the appropriate manner.
— Cyber Persistence Theory (Fischerkeller et al., 2022)
An extrapolative solution to these problems might include (1) more timely wiki updates for tradecraft guidance, (2) more research time upfront to investigate TTPs that likely work today toward a given operational outcome, and (3) more investment into tactical capability development.
Although it tends to be easier to justify incremental efforts like these, they risk misaligning with the newer fundamentals that underlie these challenges. Instead, how can we orchestrate a system in a way such that the solution to these particular challenges emerges as a natural outcome of the system’s design?
From Broadcasting to Narrowcasting
The current analogy I’m using to make sense of both the problem and solution spaces is the gradual rise of the “narrowcasting” approach that practitioners in the marketing and information operations spaces have experienced. While marketers serve content for promotional outcomes, operators serve courses of action (CoAs) toward operational outcomes. While these may seem like different fields to some, the similarities are hard to ignore. By the end of this post, my goal is to convince you that under a useful level of abstraction, these fields are indistinguishable.
An underlying theme in the evolution of any field (marketing, medicine, education, InfoSec, etc.) is the ability to perceive subtle distinctions (i.e. nuance) and the ability to manage those perceptions toward optimized decision making. If we can recognize that marketing and other fields have made more progress than commercial red teams in managing nuance, then those advancements are worth studying carefully.
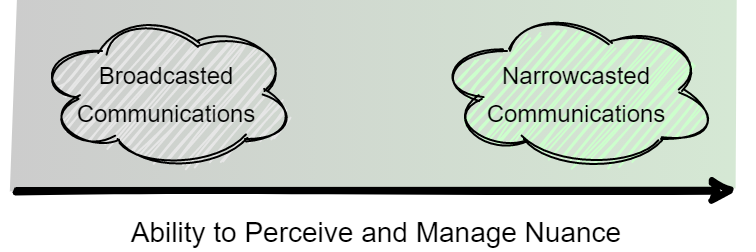
“Broadcasting” and “narrowcasting” are attributes that relate to the spread of information. For the purposes of adapting it to the context in this post, we can understand what they mean as ends of the gradient above. The more one can recognize nuance and incorporate it into their decision-making to improve the targeting and tailoring of their messages, the more they can categorize their communications as narrowcasted.
In the sections below, we’re going to further understand the gradient between broadcasting and narrowcasting from the perspectives of four interrelated properties. I’ll describe what they mean for both marketers and operators so the parallels can be recognized more clearly. The analogy should be convincing because many of us (that are adults) grew up through these changes and can relate to them.
That said, I might not be the best person to articulate this and don’t claim enough expertise. I am only articulating this because of the challenges I and others have encountered, and the desire to rediscover meaningful solutions. There’s always room for constructive criticism and improvement, and this list of properties is not exhaustive.
Anyway, the impression I get across the commercial red teams I know of is this: While most have been naturally evolving toward narrowcasted communications (whether they realize it or not), many still operate in a way that’s closer to the broadcasting side of that gradient. An explicit understanding of narrowcasting is worth serious consideration for teams that want to express tradecraft in a style that’s one notch closer to how sophisticated adversaries behave today.
Media Type: Traditional vs Modern
What I mean by traditional media (in the marketing context) includes local newspapers, radio, and television. And what I mean by modern media includes the Internet and various platforms on it like Facebook, TikTok, YouTube, Twitter, Netflix, etc.
Traditionally, media simply served as a “dumb pipe” for marketers using the conventional broadcasting approach. The technology itself did not help tailor or target the content that was served. But this is possible today with modern media—particularly with the sensemaking insights gained from the mass usage of personalized search engines and news feeds.
Similarly, we can look at “C2” software like Mythic, Cobalt Strike, NHC2, BRC4, and Sliver under that traditional broadcasting media lens. They are not designed to help tailor or target CoAs to adapt to varying operational terrain and constraints. They simply serve as the dumb pipes to convey the courses of action that were deliberated through an external process. Of course, the additional functionality I’m describing probably isn’t a common ask for now.
And if you are tracking what I’m saying over the remaining properties, you might also come to the conclusion that a publicly available operator platform and workflow that facilitates present-generation narrowcasting outcomes currently does not exist in the commercial space.
Market Segmentation: Limited vs Limitless
In the marketing context, we can think of ads being the main type of “payload” or “deliverable”, but it can also include content in a broader sense. Increasingly, there is less of a need to make a distinction. Sometimes, surrounding content can be understood as a primer for facilitating the effects that the ads are attempting to produce.
Content slots are more limited with traditional media, so ads tend to appeal to the widest possible audience eligible for the product or service being sold. The technological characteristics of those media seem to incentivize outcomes where the largest eligible market segments are prioritized. For example, a national magazine ad for a business-class ticket might be tailored for individuals who are employed in high-paying occupations, living in affluent areas, and enjoy luxury amenities.
Let’s say there’s a more specific version of that ad that’s tailored for established creatives living in Atlanta who enjoy spa treatments. The specificity in market segmentation means that it’s probably harder to justify that version for the magazine, but it makes a lot more sense to serve that ad on social media specifically to individuals matching characteristics like those. What was once a human job to match the correct deliverable for a given market segment has been cognitively offloaded to systems that can perform the matching at a scale and speed beyond human ability.
Phishing ruses have market segments too. Generic ones like payroll or benefits updates fit the broadcasting approach because they are intended to appeal to the widest possible audience and effectively have one market segment: Employee
. Success will probably be limited, but it could be further refined by tailoring it to the target company and the benefits provider (if known). This would add a couple more segments to that ruse: Employee-Company<X>-HasBenefitsProvider<Y>
.
Collecting more useful characteristics about potential targets and their environment serves as a step toward the ability to calculate which ruse (or information) might be optimally persuasive for a given target based on how susceptible they might be to its influence.
Initial access capabilities can also be understood in terms of their market segments. For example, we can think of an example capability as being suitable for the AdversarySimulation-Windows
“market”. If it’s eventually signatured by Microsoft Defender for Endpoint (MDE), then it’s targeted for the AdversarySimulation-Windows-NoMDE
market. However, a wrinkle could be discovered later on where it could still work against MDE but under limited circumstances. That would further segment its eligibility to AdversarySimulation-Windows-NoMDE-Except<X,Y>
.
The point is that it would be naïve to think that a payload could be universally “safe” or “burned”. The initiative is on the actor to recursively identify exploitable characteristics about the terrain that could further refine the eligibility of the capability. It might never become useless, but its “market segments” will narrow eligibility to a point where it will be hard for operators to humanly remember what those are every time. Much like it is impossible to humanly remember the limitless segments that represent a person when ads are being served to them on Twitter or Facebook.
And this growth in nuance points to why it’s difficult to maintain that knowledge in a wiki, runbook, or a spreadsheet. It’s because those media will never be appropriate for conveying all the wrinkles that affect the eligibility of using particular CoAs in a given situation.
Instead, a search engine could serve as alternative platform that’s more natural for narrowcasting outcomes. Its inputs may include a combination of (1) a description of the desired effect, (2) tradecraft constraints, and (3) a current snapshot of known operational terrain.
The output could convey a ranked list of CoAs to consider, where the highest ranking results are (1) in alignment with hard and soft tradecraft constraints and (2) make the most out of what is understood about the terrain (i.e. maximizing the relevant bits used to recommend a CoA).
Content Generation: “Spray and Pray” vs Tailored
In broadcasting, the way content is generated is essentially a spray-and-pray approach. You can see this in Super Bowl ads, where despite the differences in products and services, they appear to be similar overall when you zoom out and watch many of them in a row. Most of them have celebrities, use a mild sense of humour, and in recent years include more elements of nostalgia.
Similarly, many operators repeat this behaviour for initial access payloads when their content generation process consists of duct taping together common characteristics like: writing code in an obscure language, digital signatures, human-centric obfuscation, and user-mode techniques for reducing sensor observability (unhooking, direct syscalls, AMSI patching, etc.).
Both of these outcomes are characterized by the limited ability to act on nuanced information, but there is a difference in what constrains that ability. Super Bowl advertisers are limited because they are constrained by the particular broadcasting medium they’ve used for the campaign. But operators typically don’t have a hard limitation in this sense. Unlike television, cyberspace as a medium inherently offers more flexibility for content targeting and tailoring. For commercial red team operators, a major constraint is that the targeting and tailoring of CoAs (a.k.a. the content) can be time consuming to do so by hand without the aid of systems that can help recommend and construct options at scale.
A popular and relatable example of tailored content generation and targeted delivery is how Netflix personalizes their thumbnails (video explainer). They are the “most effective lever to influence a viewer’s choice” and keep them engaged on the platform. Instead of relying on the original studio artwork, creatives design dozens of thumbnails per title. Each member will see “personalized” thumbnail variants that are selected to persuade them to watch content and maximize engagement on the platform.
At this scale, it would be mind-numbingly tedious for creatives to manually search through all 86,000+ frames of an entire film or episode to produce thumbnails. So there is a system in place to recommend frames that could be viable candidates for thumbnails. This makes the process more triage-based and allows them to apply their intuitions and expertise in a more sustainable manner. With a pool of thumbnails available per title, the platform essentially performs pattern matching to rank each thumbnail by the influence the thumbnail could have on the member playing the title. The highest ranking thumbnail is what the member sees.
The optimal assignment of image artwork to a member is a selection problem to find the best candidate image from a title’s pool of available images. … The model predicts the probability of play for a given image in a given a member context. We sort a candidate set of images by these probabilities and pick the one with the highest probability. That is the image we present to that particular member.
— Artwork Personalization at Netflix (Netflix Tech Blog, 2017)
All of this to say that we can think of the evolution of red team capability development and CoA delivery processes in a similar narrowcasting lens, while being mindful of the differences in stakes and speed. As demonstrated in the Netflix example, it’s not hard to imagine how recommendation systems could play a role in tailoring the development of tactical capabilities as well for targeting to determine eligibility against a variety of terrain and constraints.
As with thumbnail development, tactical capability development could also have triage-based workflows driven by observed updates to defensive products as well as gaps in operators’ search results for options that align with their terrain and tradecraft constraints. These workflows would help narrow down the search space and help developers apply their intuitions and expertise more productively. This can compliment creative, unstructured processes in R&D (not replace).
At the time of writing, it would not make sense for commercial red teams to completely automate the selection of CoAs at the scale that Netflix’s platform automatically selects thumbnails. Serving the wrong CoA is usually more consequential than serving the wrong thumbnail, and the difference in stakes and speed will shape which decision-making models are appropriate for the situation:
- Human Is The Loop: Only humans are involved in analysis and decision making.
- Machine Is The Loop: Only machines are involved in analysis and decision making.
- Human In The Loop: Humans receive cues from machine analysis to make decisions.
- Human On The Loop: Humans supervise machines; human decisions are more meta.
Worth noting that the definitions above have been simplified for the purpose of this post. Below are visual descriptions for each of the models as they relate to selecting persuasive Netflix thumbnails or persuasive payloads. Take a moment to look at the graphics and evaluate how well each model would fit with the use case. The main takeaway here is to recognize that the served content is domain-agnostic and that selecting an appropriate model depends on the use case.
It’s not clear to me how useful the Human On The Loop model would be for the decision spaces or channels that are currently popular for consulting red teams. But loop position aside, there is still value in the calculated ranking of CoAs and the accumulation of interactions an operator could have with this type of “in the loop” platform. It would serve as useful context to regularly challenge their intuitions and guide their decision making in a rapidly changing landscape.
Almost all of the publicly-available “C2” platforms in the commercial red team space are missing this functionality because they are based on the Human Is The Loop decision-making model, which was more common in the traditional broadcasting paradigm. The sheer volume of information available to target and tailor communications in the narrowcasting paradigm makes it impractical to have human-only analysis processes.
Overall, the tailoring and targeting of content that’s associated with narrowcasting is a departure from the traditional spray-and-pray or “duct-tape-all-the-evasion-techniques” approach used in the broadcasting model. At a useful level of abstraction, we can learn a lot from how Netflix tailors and targets thumbnails—even though the content being served is distant from the content that operators serve.
Information Resonance: Lower vs Higher
This next property is about how well the communicated information could resonate with its audience, in pursuit of the effect the marketer or operator is attempting to produce. When watching a live broadcast stream (news, sporting events, etc.), the ads between the content will probably resonate less than the personalized ads one might see on their Twitter or Facebook feed.
There’s a particular analogy I like to use to further describe what I mean by resonance. In acknowledging its limitations, the least convoluted version of it goes like this:
Imagine a series of rooms where the goal for each room is to get from one side to another. But there’s a twist, invisible laser tripwires are placed all over and one must carefully dance around them to succeed. The tripwires in each room will vary in capability and positioning.
So let’s analyze this from multiple contexts. Some of the language might sound a little strange from the marketing and red team contexts, but that’s also the point of using an analogy. (If you’re looking for a visual that’s close enough, watch this scene from Ocean’s Twelve.)
Abstract Context
Each room represents an environment where messaging must be constrained (or shaped) to maximize a desired outcome. The dance is the message, and each dance move serves as a lever to shape that message. Each tripwire represents an ability to perceive some aspect of incoming information that undermines the system’s survival.
To adapt and evolve, there’s the incentive to have many diverse levers to flexibly shape messages. The fact that the tripwires cannot be visibly observed indicates another incentive to construct the goggles that recover their capabilities and configurations. For the purposes of this analogy, a message’s resonance is the degree to which it is shaped to avoid triggering undesired perceptions.
Marketing Context
When a Super Bowl ad is broadcasted to millions of viewers, we can think about that as simultaneous “laser dances” across millions of “rooms”. Each room serves as the environment of an individual viewer. The “tripwires” within each room serve as the mental frames or mental models they can use to parse reality and detect unwanted information—often automatically. One can think of the selected “dance moves” as coming from a pool of various methods that can be used to produce the ad (celebrities, humor styles, image saturation levels, shot frequency, etc.).
With differently configured tripwires in each room, it’s easy to see why a broadcasting approach produces lower information resonance. Limitations in broadcast technology mean that if the same “dance” has to be performed in each room, it can only be choreographed to avoid what marketers perceive as the most common tripwires found across most rooms.
With technology suitable for narrowcasting, marketers can start to interact with individual rooms. With that comes the dual ability to “recover” individual tripwires and use that knowledge to design well-choreographed dances at a per-room level. In other words, the information sent under a narrowcasting lens is a tailored response to the frames each recipient is likely to activate for perceiving changes in their environment.
Operational Context
Similar dynamics play out in cyber operations. Each “room” can be flexibly understood as the terrain relevant to producing a particular effect. Effects could be defined at different scales (tactical to strategic, i.e. fractal). Each “laser dance” is the communication sent to produce an effect (e.g. packets sent over a network), and each “dance move” is selected from a pool of configurable procedures to shape the communication. The “tripwires” within each room equate to the various procedures embodied in defensive sensors to parse changes in the operational terrain.
To ground this with an example, we can think of process injection as a tactical effect being produced. The room (or relevant environment) is the endpoint where the effect would occur. The dance (or communication) is the byte sequence sent from the operator platform to the implant. The dance moves (levers) include a variety of injection procedures that can be configured in different ways to shape the bytes sent to the implant. The tripwires are the pool of defensive procedures (e.g. within an EDR) that can detect the CoA.
The goal then is to shape the byte sequence to produce the desired effect, without triggering any tripwires. How the bytes are shaped depends on the level of nuance understood and the ability to manage it.
On one end of this gradient, the broadcasting approach can be characterized by developers focusing their time and efforts on building a versatile payload that should work in most environments. Much like marketers working on a Super Bowl ad that should appeal to most audiences. Development is driven by an anecdotal understanding of past successes and failures that is primarily oriented around how the developers shaped the communication. How the defensive sensor perceived the communication tends to be an afterthought—if considered at all.
On the other end of the gradient, the narrowcasting approach is characterized by developers who would proactively know what kind of tripwires are likely to be triggered and what the procedural logic for each would look like. Crucially, the data can be used to shape those bytes in ways that are highly situational and in ways that can sometimes challenge developer intuitions. Much like in the marketing context, the communication becomes a tailored response to the procedures that relevant defensive sensors are likely to activate for perceiving changes in their environment.
Considering that the tripwires are invisible, more investment goes into building the goggles to observe them. Building those helps with efforts to model specific rooms and simulate the dances within them in useful ways. This approach effectively produces outcomes where tradecraft expressions are diverse by default, and the concept of a universal payload becomes less useful.
Today, the difference in the commercial red team scene (not everywhere, but perhaps overall) is that a lot of effort goes into developing the “dance moves” if you will, and not enough into proactively and precisely mapping out the “tripwires”. The systematized mining of tripwires allows for more nuance in engagements and is a key component in effective recommendation systems.
As an aside, when the tripwires are not understood precisely enough, it consistently leads to a lot of misleading blanket statements on InfoSec/RT Twitter about what’s OpSec-safe and what isn’t. Low-nuance content tends to get more engagement, and the platform itself seems to be algorithmically incentivized to elevate it. This positive feedback loop effectively rewards (at scale) a style of tradecraft that’s closer to broadcasting than it is to narrowcasting. Anecdotally, the more I spent time outside of Twitter, the more that helped me understand the narrowcasting paradigm.
To recap this analogy, we can (crudely) summarize the difference in operational styles as follows:
Broadcasting
- C2 platform serves as a “dumb pipe” to deliver externally deliberated CoAs.
- Human operators’ anecdotes and intuitions primarily drive CoA selection.
- Evasion is the duct taping of syscalls, unhooking, and other undeniable procedures.
- Tooling leans more toward universal “dance moves”, and less on mapping “tripwires”.
- Tactical tooling development is prioritized in anecdotal and reactive ways (headwind).
- CoAs tend to cluster around what anecdotally worked well before.
- Versatile tactical tooling drives success.
Narrowcasting
- C2 platform not only delivers CoAs, but also helps with tailoring and targeting.
- Recommendation systems calculate CoA options, operators help cross-check.
- Communications are tailored responses to anticipated defensive sensor procedures.
- Modeling and simulation plays as much of a role as tactical tooling does.
- Data-driven workflows proactively organize tactical tooling development (tailwind).
- CoAs tend to appear amorphous by default.
- Superior information drives success.
If you participate in commercial red team ops, where does your approach fall on this spectrum?
Applications for Optimized Recommendations
If you see the merits in tracking tradecraft evolution along the spectrum from broadcasting to narrowcasting, you might also be thinking about the implications on what it would actually take for defenders to detect sophisticated adversaries, and what it would take for red teams to overlap with realistic adversary activity—especially when the overall conversation on both sides has been so TTP oriented. You might also notice that popular Twitter discourse, public tooling, and training offerings from SANS and OffSec currently do not reflect the narrowcasting part of this recap.
Indeed, some of those outcomes seem somewhat distant today for many commercial red teams, and yet we can see they’re not so distant for marketing and tech companies. That’s because one aspect of what makes narrowcasting outcomes more viable for them is the concept and use of recommendation systems (RecSys).
Earlier, we discussed how Netflix uses them in both the tailoring and targeting of their thumbnails. That is just one example among many we see the outcomes of every day. More examples include: scrollable content feeds on social media (Twitter, YouTube, TikTok, etc.), product suggestions on Amazon, navigation plans in Google Maps, and ads.
Despite their popularity, it’s not as accessible to learn how these systems work in production:
Recommendation Systems (RecSys) comprise a large footprint of production-deployed AI today, but you might not know it from looking at Github. Unlike areas like Vision and NLP, much of the ongoing innovation and development in RecSys is behind closed company doors. For academic researchers studying these techniques or companies building personalized user experiences, the field is far from democratized.
— Introducing TorchRec (Meta AI, 2022)
In this section, we can try to expand the public discourse of red team workflows and tooling by discussing the high-level merits of recommendation systems.
It’s worth reminding that what it takes to implement present-generation capabilities that facilitate narrowcasted communications has very little to do with what it takes to build “malware” and “C2”, and yet has a lot to do with how they’re used. In a similar line of thinking, the skills required to build Enigma-decrypting computers in WWII had little overlap with the skills required to build artillery used on the battlefield. Yet the careful analysis of the decrypted messages determined how the artillery was used.
We can compare the RecSys architectures in YouTube and TikTok as a starting point to see how what is useful for ad-tech companies could also be useful for red teams (and advanced actors). We won’t go into specific implementations here as I have more questions than answers at this point, so this discussion will be conceptual to surface the value prop for commercial red teams.
Recommendations in YouTube
There is a 2016 paper that goes over YouTube’s recommendation system at a high-level, and the authors include a diagram (shown below) outlining the key components in its architecture. The home page of the YouTube app will present the outputs of this system: a ranked list of videos.
Going from left to right, we start with a corpus of millions of videos which are filtered through two stages. First, the “candidate generation” stage uses information about the user’s recent activity on the platform (e.g. views, likes, comments) as well as context (e.g. user demographics, time of day). Second, the “ranking” stage assigns a score to each video according to a desired objective function using many features describing the video and user. Lastly, the videos are presented to the user ranked from highest to lowest.
In order for a system like this to function, we can infer more requirements: The corpus of options should be organized in a way where the metadata is machine-readable. There should be real-time telemetry of how the users are interacting with the platform and what they are able to observe in their environment. With the incoming telemetry for options, user behavior, and environmental observations, systems should be assigning characteristics about them for later decision making.
With this understanding, we can now try to apply this model for tactical CoA recommendations. (While recognizing the potential for CoA recommenders at operational or strategic levels.)
Going from left to right again, we start with a corpus that consists of procedural options which are filtered through the same stages. You can loosely think of this as your database of TTPs.
The “candidate generation” stage filters options in a way to get what’s relevant for the desired tactical effect, as well as what’s possible within what’s currently known of the operational terrain. This version of the model introduces search queries for goal-oriented recommendations, whereas the recommender on the YouTube home page surfaces what they call unarticulated wants.
After those candidates are generated, the “ranking” stage assigns scores to them with an objective function that determines how the characteristics of each candidate option matches with the desired tradecraft constraints of the operation. The difference is that instead of maximizing user engagement, it’s more about maximizing compliance to the operation’s tradecraft constraints.
So what we could get out of this is something that roughly looks like a Google search engine. By entering a query like “process injection”, we can get a list of recommendations that have been filtered by what’s possible given what’s known about the operational terrain, and then ranked by tradecraft constraints (e.g. based on engagement type).
It’s interesting to think about the time this would save, particularly in relation to the second challenge of expensive human decisions being used to counter cheap machine decisions. Manually discovering and evaluating each of these recommendations from the diagram takes time and cognitive effort, and that’s where this type of automation could help.
To take the point further, when you think about AI—not as specific algorithms—but as an outcome where some of that previous cognitive effort is now offloaded, that’s what this is a step toward. Similarly, if you want to understand an operation well, you focus on the outcome and the follow-on effects that can come from it. In this view, both are less about a play-by-play of the specific “TTPs”, which can be fungible to a degree.
Recommendations in TikTok
There is a 2020 article that attempts to interpret how Toutiao’s recommendation system works with the conjecture that TikTok has a similar architecture because ByteDance is the parent company of both.
The article describes the slide above as follows:
A recommendation system, described in a formal way, is a function that matches users’ satification with the served content using three input variables:
Xi
,Xu
, andXc
.
Xi
represents content, including graphics, videos, Q&As, major headlines. It’s imperative to consider how to extract characteristics of different contents to make recommendations.
Xu
represents to user characterization. It includes various profiles such as interest tags, age, gender, occupation, etc, in addition to implicit user interests determined by user behavior models.
Xc
represents environment characterization. This feature is an artifact from modern mobile Internet, where users can move between different locations such as home, commuting, work, travel destinations, etc.Combining all three input variables, the model can provide an estimate of wheter the recommendated content is appropriate for the user in a given scenario.
With this understanding, we can perform a similar thought exercise that tries to crudely map this high-level model to serve tactical CoA recommendations instead of entertainment content:
-
Xi
represents tailored communication content across all information channels (targeting both humans and machines). It’s imperative to consider how to extract characteristics of different contents to make recommendations. -
Xu
represents to tradecraft characterization. It may include various dispositions in the form of hard and soft constraints in addition to relevant behavioural characteristics inferred from specific threat actors. -
Xc
represents terrain characterization. For example, in the endpoint context, this can include information about defensive sensor products and their configurations, as well as information about installed programs. These can be used to surface opportunities that are specific to this environment.
Combining all three input variables, the model should provide an estimate of whether the recommended content is appropriate for the operation, given what is known about the terrain.
Recommendations in Defence
Now, of course, these comparisons with TikTok and YouTube were not arbitrary thought exercises. Much like the narrowcasting analogy was not arbitrarily chosen. Adversaries also understand the importance of systems like these, and so should any commercial red team that is inclined to gain a deeper understanding of sophisticated adversaries (beyond the TTPs they were caught using).
“Ad-tech will become natsec-tech,” as stated in the 2021 final report by the National Security Commission on Artificial Intelligence (NSCAI). Although this report is intended for policymakers, I’d recommend reading at least the executive summary and first chapter to help build a shared high-level understanding of what AI-enabled cyber operations means. A couple quotes follow:
Eric Schmidt, the co-chair of the commission and former CEO of Google summarized it well (although the use of future tense intuitively seems misleading):
AI is going to be used as part of cyber conflict (active and defensive strategies will all be AI-based). And AI will be used to increase targeting. In other words, "the missles" if you will, will become more accurate and quicker because of this technology. Some of this is part of a normal evolution of greater accuracy [and] precision in how our military works.
— Eric Schmidt (War on the Rocks Podcast, 2021)
For meaningful reasons, it’s difficult to get a sense of what the implementation of these systems actually look like. But the emphasized slides below provide some hints on how AI-enabled capabilities are becoming the norm for CoA analysis and decision-making. This makes sense given that the “Human Is The Loop” model is becoming less competitive in a growing number of decision spaces.
Keep in mind that much of the context in these documents is naturally lost from my limited perspective. There are also methodological gaps when focusing solely on the US perspective in the development of these capabilities.
Efforts like these should not be news to commercial red teams that are trying to understand how sophisticated adversaries function on the inside. But if these aspects were widely understood, common discourse around popular topics like “EDR evasion” would use language that fits more with the narrowcasting paradigm.
It is appropriate to be skeptical of the evasive abilities certain “Human Is The Loop” C2 platforms advertise, when their own language indicates they’ve put more focus on versatile “dance moves” instead of the adaptive capabilities that precisely respond to specific and situational “tripwires”.
This concludes the discussion of the narrowcasting analogy and how it might be operationalized. Because this style of operating hasn’t already been common for many red teams, the next section attempts to describe a way to think about what constrains our understanding of apex adversaries. I don’t know if it will be convincing, so consider it as speculative food for thought and further discussion.
Misaligned Incentive Structures
-
Why are AI-enabled red team operations not already commonplace? Why are commercial operators still using “Human Is The Loop” decision-making models which give rise to the three challenges described at the beginning of this post? Are we hiring and training for the appropriate skillsets to develop and correctly use “Human In The Loop” platforms? What outcome does the status quo serve?
-
Are enough defenders adapting to the AI-enabled nature of adversary operations? How much confidence can analysts put into defensive telemetry when threat actors can precisely respond to situational tripwires using recommendation systems that improve the targeting and tailoring of their CoAs? What are the more subtle threats that might be overlooked in favour of the big-ticket “instant” threats that executives seemingly need to care about?
These are two sets of questions I’ve been asking myself lately. Parts of commercial InfoSec will always lag behind the state-of-the-art when the funding and stakes aren’t high enough. That said, the extensive use of recommendation systems and related technologies in commercial red teaming appears to have been delayed by nearly a decade, compared to when the effects of those systems were popularized in many other verticals.
There can be many ways to answer these questions and it’s hard to come up with a simple answer that isn’t overly reductive. My overall view is that there are myopic and self-reinforcing incentive structures in the commercial space that slow down our ability to make (or even recognize) leaps in sophistication—unless they can be positively rewarded by market forces. Reward has a specific meaning which we’ll circle back to.
This suggests (perhaps paradoxically) that despite the rising budgets security vendors and in-house teams have been accumulating, the pursuit of rediscovering sophistication leaps in adversary capabilities fails to match pace, and in turn, so does our ability to defend against them.
Although this suggestion lacks thorough analysis (and might state the obvious to some), we can discuss two lenses to build an intuition for how it unfolds. And of course, “all models are wrong, but some are useful” continues to be a relevant aphorism.
If the suggestion is true, this is an unintended consequence, and perhaps the sociological lens of latent functions would be more fitting. A “latent function” of a behaviour is not announced, not perceived, and not intended by the people involved. Contrast that with a “manifest function” of a behaviour which is explicitly announced, recognized, and intended by the people involved. This is somewhat similar to effects-based thinking which is also a framework for understanding the consequences of actions.
Another lens relevant to interpreting this suggestion is reinforcement learning, as described by Sutton and Barto. Informally speaking, “agents” exchange signals with the “environment”. The agents emit “choice signals” to the environment, and the environment emits back “reward signals” that inform future choices. The word “choice” suggests that each agent has a purpose. The agent’s purpose is to maximize the total amount of reward it receives. The agent will always learn to maximize its reward. The reward signal tells the agent what should be achieved, not how.
Putting the two lenses together, we can now articulate the suggestion as follows:
-
The agents represent a variety of choice-making practitioners in commercial InfoSec. This includes people who take on roles such as behaviour discrimination (DFIR, CTI, MDR, etc.), behaviour generation (pentesters, red teams, etc.), executive leadership, vendor sales, vendor marketing, and more. The environment conceptually represents the market.
-
Practitioners learn to make choices that maximize the reward signal emitted back from the market. Financial reward signals come in the form of expected revenue or loss reduction. Social reward signals come in the form of engagement (views, likes, shares, reactions, etc.). These signals inform future choices as they tell practitioners what should be achieved.
-
A latent function forms where there is friction in our ability to understand and defend against adversarial adaptations of narrowcasting dynamics. This is not explicitly stated, recognized, or intended by most practitioners involved. The behaviour from which this function emerges is the aggregation of practitioners maximizing their reward.
* * *
Another latent function that seems to occur from the aggregate maximization of reward is myopia in recognizing the extent of how adversaries may interact with enterprise environments. We can frame this problem with two specific questions that aren’t acknowledged enough in some circles:
-
In commercial environments, we often threat model and red team scenarios that directly and linearly undermine company prosperity (compliance fines, theft, etc.). But how might threat actors “interact” with a company’s information technology in ways that can appear to make the company more profitable?
-
Will the company’s leadership be motivated to prioritize the reduction of these scenarios before the second-order effects of those interactions become widely perceptible?
It’s likely that in many circumstances an answer to the first question wouldn’t be obvious, and getting an answer to the second question might be met with friction and reluctance.
Perhaps the most recognizable demonstration of this latent function was the naïveté of particular social media companies during the early 2010s. Targeting features that could be used to optimize courses of action for red team operations, are used today to calculate the unarticulated wants of individual social media users which places them into personalized filter bubbles.
The first-order effect of this is that it keeps users engaged which in turn maximizes revenue (reward). The second-order effect is the quiet erosion of our collective ability to build consensus in society and have a shared sense of reality. Furthermore, what keeps users engaged the most is outrage. This constructs a situation where published content that serves adversary interests is poised to amplify the second-order effect.
Social media companies are becoming aware of their role in the problem, but not all Silicon Valley leaders are convinced of their responsibility to eliminate false news. Fighting spam is a business necessity, but terminating accounts or checking content constrains profitability. Social media companies have a philosophical commitment to the open sharing of information, and many have a limited understanding of the world of intelligence operations.
— Who Said What? The Security Challenges of Modern Disinformation (CSIS Academic Outreach, 2018)
Adversary interactions with social media infrastructure is just one example, and it’s worth exploring what this type of profitable interaction looks like in other verticals. Critically thinking about an adversary’s strategic efforts and the two questions above could help in that direction.
Now of course, it seems reasonable to question why sufficient effort should be placed into surfacing the behaviour of adversaries that are operating through an enterprise and not directly against it—especially when threats like ransomware are more clear-cut and demanding. After all, enterprise security is not supposed to share the responsibilities of national security.
This separation of responsibilities is a uniquely Western notion, and those responsibilities tend to be intertwined in other competing countries. We should not underestimate how competitive they can be when they can organize a command and control situation to share those responsibilities.
Takeaways
The purpose of this post was to (1) surface some fundamental gaps many commercial red teams appear to have in their tradecraft and capabilities for apex adversary simulation, (2) discuss the merits of recommendation systems for red team workflows and tooling, and (3) describe what makes the commercial space slow to defend against advances in apex adversary workflows.
-
The narrowcasting analogy continues to serve as a useful (albeit abstract) mental model to inform the evolution of red team tradecraft and capabilities. In the absence of reliable access to threat actor workflows and capabilities, the model provides fertile ground for understanding both the problem and solution spaces in our domain.
-
Ad-tech is indeed natsec-tech. We should draw inspiration from programmatic advertising to boost our ability to target and tailor our courses of action in operations. Although this is different from the tactical systems and infrastructure programming that currently permeates discourse in the commercial red team space, it is arguably just as consequential.
-
What are the choices we make in the commercial space that maximizes market reward signals, while simulatenously distorting our ability to recognize and react to the extent of apex adversary intentions and capabilities?
Thank you for taking the time to read this, and I hope there is at least something you found useful. I hope it helps stimulate thinking, discussion, and even differences of opinion so we can evolve the quality of the discourse and ultimately improve defence.
And thank you to those who provided feedback on earlier drafts!
If you found this post intuitive, you might like: